基于深度学习的两类典型语音处理问题研究
更新时间:2023-05-08 13:03:01 阅读量:2 实用文档 文档下载
- 深度学习算法推荐度:
- 相关推荐
国防科学技术大学研究生院硕士学位论文
ABSTRACT
Deep learning is one of the most advanced research fields in artificial intelligence, and it has made astonishing progress in computer vision, speech processing, robot control, and bioinformatics. Deep learning conducts analysis and learning in a way of simulating human brain, and generates complex concepts by abstracting and combining simple concepts. Comparing with conventional machine learning algorithms, deep learning does not extract hand-crafted features.
In this thesis, we studied two typical deep learning based application problems in speech processing, namely audio matching and audio visual speech recognition. From the viewpoint of engineering, audio matching and speech recognition are key technologies of speech processing, and have been widely used in speech retrieval and intelligence analysis. From the viewpoint of theoretical study, audio matching and speech recognition are typical unsupervised problem and supervised problem in speech processing, respectively. Researches on deep learning models for these two kinds of problems are of great academic value. There are following major contributions: First, to improve the generalization capabilities of traditional audio matching methods, this thesis proposed to extract audio features via Convolutional Deep Belief Networks (CDBNs). CDBNs combine advantages of Convolutional Neural Networks (CNNs) which deal with high dimensional data and those of Deep Belief Networks (DBNs) that conduct unsupervised learning, and can extract features with strong generalization capabilities from high dimensional audio data in an unsupervised way. Based on the binary features extracted by CDBN, we proposed a faster audio feature matching algorithm. Experimental results show that CDBN based audio matching algorithm significantly improves the hit rate of audio matching, compared with traditional chroma energy normalized statistics feature based audio matching algorithm.
Second, to integrate both temporal characteristics of audio information and video information, this thesis proposed a multimodal Recurrent Neural Network (RNN) framework for multimodal speech recognition. The framework consists of an auditory part for processing audio data, a visual part for processing video data, and a fusion part for combining both the auditory and visual parts. The experimental results demonstrate that the proposed speech recognition system based on multimodal RNN successfully combines video features and audio features, and effectively improves speech recognition accuracy based on audio data only, especially on the low SNR dataset.
Key Words:Deep learning, speech processing, audio matching, audio visual speech recognition
第ii 页
国防科学技术大学研究生院硕士学位论文
第 iii 页 英文缩写词对照表
CDBN
卷积深度置信网络(Convolutional Deep Belief Network) CENS
色度能量归一化统计(Chroma Energy Normalized Statistics) RNN
递归神经网络(Recurrent Neural Network) DNN
深度神经网络(Deep Neural Network) SGD
随机梯度下降(Stochastic Gradient Descent) ReLU
修正线性单元(Rectified Linear Unit) BP
反向传播(Back propagation) LSTM
长短时记忆(Long Short Term Memory) GMM
高斯混合模型(Gaussian Mixture Model) HMM
隐马尔科夫模型(Hidden Markov Model) DBN
深度置信网络(Deep Belief Network) CNN
卷积神经网络(Convolutional Neural Network) RBM
受限玻尔兹曼机(Restricted Boltzmann Machine) CRBM
卷积受限玻尔兹曼机(Convolutional Restricted Boltzmann Machine) AVSR
听觉-视觉语音识别(Audio-Visual Speech Recognition)
国防科学技术大学研究生院硕士学位论文
第iv 页
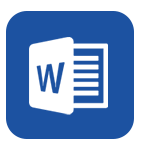





正在阅读:
基于深度学习的两类典型语音处理问题研究05-08
2015年国产陆地观测卫星绝对辐射定标系数(CBERS04GF2GF1ZY3ZY102CSJ9AHJ1AB)09-18
homework-106-20
2016年监理工程师(机电安装工程)延续注册考试题及答案(82分)05-22
微机接口实验8251 北工大 - 图文04-01
弹性硅pu地坪施工合同06-20
翻译03-15
马克&183;吐温简介06-08
加盟药店的管理办法01-28
商业地产项目(汽配城)招商人员标准问答术05-25
- 教学能力大赛决赛获奖-教学实施报告-(完整图文版)
- 互联网+数据中心行业分析报告
- 2017上海杨浦区高三一模数学试题及答案
- 招商部差旅接待管理制度(4-25)
- 学生游玩安全注意事项
- 学生信息管理系统(文档模板供参考)
- 叉车门架有限元分析及系统设计
- 2014帮助残疾人志愿者服务情况记录
- 叶绿体中色素的提取和分离实验
- 中国食物成分表2020年最新权威完整改进版
- 推动国土资源领域生态文明建设
- 给水管道冲洗和消毒记录
- 计算机软件专业自我评价
- 高中数学必修1-5知识点归纳
- 2018-2022年中国第五代移动通信技术(5G)产业深度分析及发展前景研究报告发展趋势(目录)
- 生产车间巡查制度
- 2018版中国光热发电行业深度研究报告目录
- (通用)2019年中考数学总复习 第一章 第四节 数的开方与二次根式课件
- 2017_2018学年高中语文第二单元第4课说数课件粤教版
- 上市新药Lumateperone(卢美哌隆)合成检索总结报告
- 深度
- 语音
- 典型
- 基于
- 处理
- 研究
- 学习
- 问题
- 2020年内蒙古自治区《病理学》模拟卷(第913套)
- 毕业论文过程检查总结doc
- 八年级下册英语第二单元知识点指导:课文解释
- 人教版八年级上册生物541细菌和真菌的分布同步测试
- 科技小论文的撰写方法
- 浙江省嘉兴市汽车及零配件批发行业企业名录2019版355家
- 《梦想不会辜负努力的你》读后感700字_读后感_模板
- 初中自我评价800字参考范文五篇
- Excel函数大全【超全】
- 原告陈明东与被告王振离婚纠纷一案一审民事判决书
- 五年级的语文一对一学习的教案.doc
- 水库承包合同(完整版)
- 高中地理必修一同步练习:1.1《宇宙中的地球》1 Word版含答案
- 2016年计算机二级《ACCESS》模拟基本操作题及答案
- 浅析市心街历史街区改造项目定位规划和开发建设
- 西方经济学模拟题及参考答案(共6套)
- 六年级下册英语试题Unit 1 The lion and the mouse Story time译林版(含答案)
- 人教版二年级数学下册千以内数的认识100
- 哈药集团2010年度股东大会决议
- 2017年大学生士兵提干考试基本常识:盘点“墨子号”量子科学实验卫星四大任务